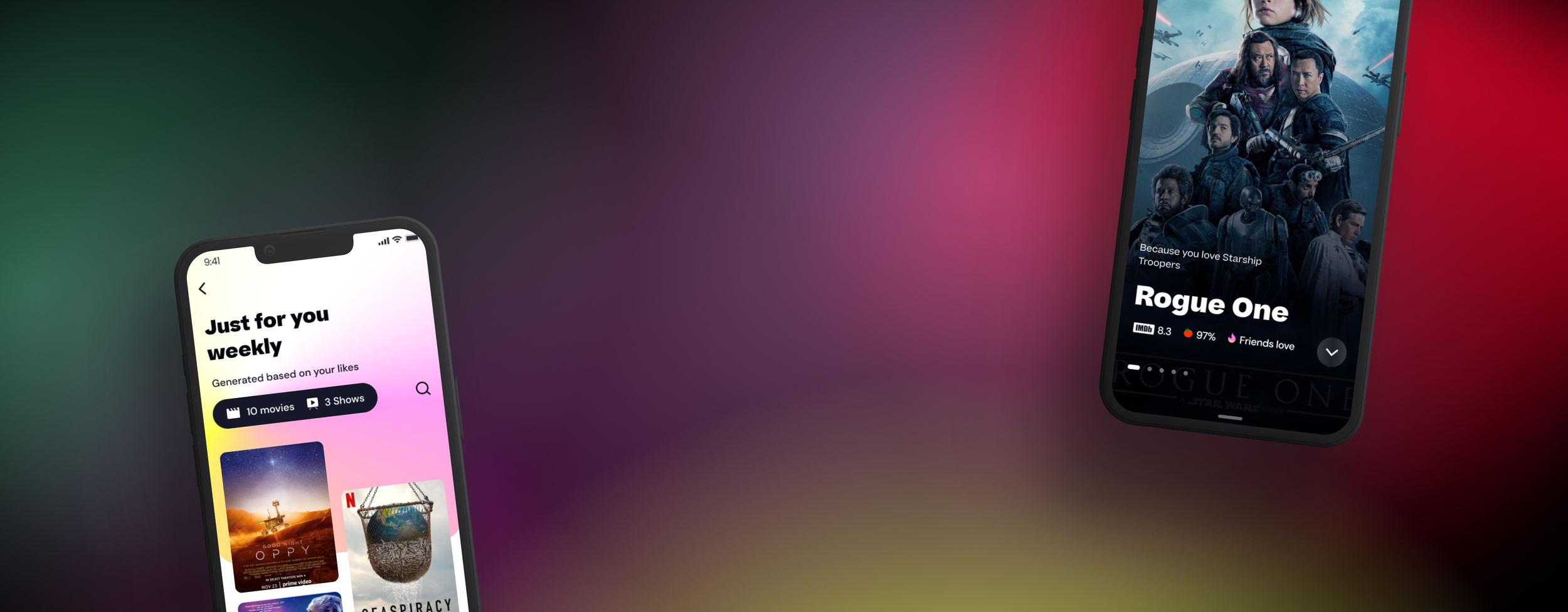
Reimagining content discovery
SUMMARY
We pioneered a vision and proof of concept for a top UK telecoms and broadcasting company, transforming content delivery, aggregation, and recommendations. Our unique, low-risk solution directly catered to their customers' needs, with significant potential to enhance the core business.
To do this we’d leverage the client’s unfair advantages, such as its large customer base, its deep understanding of customer behaviour, and its extensive content library.
OUTCOME
A visionary design concept and separate GPT-4 powered prototype forming a validated opportunity for our client to
Engage their existing customer base
Develop new income streams
Generate an attractive cost per lead
TYPE
Validation sprint (product vision and market fit) and engineering POC
TIMELINE
Senior Experience Designer
Skills:
User research
User testing
Workshop facilitation
Lo-fi mock-ups
Figma prototyping
Stakeholder management
ROLE & SKILLS
Lead Experience Designer
Senior Strategic Designer
Senior Experience Designer
2 x Lead Engineer
Product Manager
General Manager
Venture Architect
TEAM
OBJECTIVE
Develop a vision and prototype for a content recommendation engine that will address existing pain-points and provide customers with a more personalised and engaging content discovery experience.
Project Overview
The overall project set-up was designed to move rapidly and cover multiple rounds of design research, growth tests and engineering a proof of concept
PHASE 1 - DISCOVERY - 4 WEEKS
Design
Understand the content decision-making context and identify pain points for potential Novi features in one testing round
Build initial hypotheses and test/ de-risk
Growth
Launch growth test to quantify design research hypotheses and prioritise value props and initial features
Engineering
Research data accessibility and build options for POC, mirroring requirements for Novi compatibility with VMO2 infrastructure
PHASE 2 - DESIGN AND BUILD - 6 WEEKS
Design and test value props and features
Design
Test converged set of propositions and features in two testing rounds, and layer on survey, prototype and growth results
Growth
Launch growth tests focused on gaining quantitative data on narrowed value props and features via different channels
Engineering
On-going de-risking of build components and build coded POC focused on key functionality around prioritised components
Converge to final solution vision and proof of concept
PHASE 1 - DISCOVERY
Learning how people watch
During the first phase of the project, we uncovered key pain points, identified behavioural archetypes and prioritised early desirability.
To remove biases, we used a mix of quantitative and qualitative methods including surveys, remote and in-home interviews, and growth tests on social media.
25+
Early propositions and features tested
30+
Hours spent with users
600+
Respondents from survey
A common pain point
The primary user pain point that formed the foundation for the validation sprint was that users spend more time searching for content than enjoying it. Looking a little deeper, we found it had three core components:
Three behavioural archetypes
Analysing our testing outcomes, we also identified 3 distinct behavioural archetypes, each with their own individual needs, pain points and associated features.
PHASE 2
Uncovering valued features and propositions
Taking from the refined list of feature ideas we’d discovered in the previous round, I developed basic wireframe mock-ups of each in preparation for our second round of user research. This round, with 10 participants, specifically looked into which features and value propositions resonated the most.
I created user research boards with mock-ups of the individual features to help illustrate their functionality to participants
Testing Value Propositions
We also created mock landing pages for three distinct value propositions, each containing a mix of different features for testing participants’ preferences. We wanted to gauge what people gravitated towards most strongly.
Synthesising our research into user needs
Having carried out the 10 qualitative interviews, it was clear the themes people were interested in:
Advanced filtering and search
Advanced algorithms and mechanisms that dynamically understand user preferences, behaviours, and context to deliver highly personalised and relevant content suggestions
Content aggregation and curation
Systematically gathering diverse content from various sources and then meticulously selecting, organising, and presenting it in a coherent and meaningful way
Smarter group watching
Employs collaborative algorithms to suggest content that aligns with the collective preferences of a group watching together
Synthesis of user research round 2
Underlying user needs
We distilled the outcomes of our testing efforts into three fundamental user needs, consolidating valuable insights to drive our strategy.
FINAL TESTING ROUND
Developing realistic mock-ups for final testing
For our final testing phase, our aim was to present realistic visuals to our test participants and provide a more authentic visual experience. The purpose of this round was to fine-tune any aspects that required adjustments before finalising the solution. This step was pivotal in guaranteeing that our product not only functioned seamlessly but also resonated effectively with our users.
Higher fidelity mock-ups for round three of testing - we wanted to understand how participants reacted to individual elements and features
The final prototype: a vision for content recommendation
The screens below represent the final Figma prototype of the overall vision we presented to the client, the result of three rounds of research and 6 weeks of rapid design. The engineered GPT-4 POC was delivered separately.
Onboarding that learns your likes
With a quick swipe, Novi will begin to learn the user’s likes and dislikes, ensuring that upon arriving at the first set of recommendations, they are relatively accurate. Over time, the algorithm will learn and adjust to suit the user’s preferences.
Mood-based recommendations
Novi will curate a homepage personalised for every user. With a quick tap they can search for content by mood - in this case, for something uplifting. Novi searches across all streaming platforms and peppers in some generative AI to arrive at a suggestion.
Seamless viewing experience
Keen to learn a little more about the movie? Novi aggregates reviews from Rotten Tomatoes, IMDB and friends’ profiles to provide expert reassurance and social proof. Saving time checking across the platforms, Novi has already chosen the cheapest method to watch. Meanwhile, the user’s TV has been detected and, in a playful way, the movie can be “flicked” to the TV to play.
Tune recommendations your way
As user tastes evolve over time, Novi allows editing of the algorithm both indirectly and, as shown here, directly. Our research showed that users want recommendations to be more transparent - not the typical black box.
Curated spaces
With curated spaces, users can create their own, or let Novi recommend some - for example ‘Just for you weekly’, a finely curated weekly collection based on viewing habits and tastes. These ‘playlists’ can be shared with friends and joined into group spaces, so there’s always something to watch with others.
Group voting
During movie night, instead of the usual argument, try GroupVote, a fun way to decide what to watch.
Impact
Novi showcases the synergy between user-centered design and advanced technology. Its AI-based content recommendation system has performed exceptionally, earning significant praise and interest from our client.
As a result, plans are underway to further develop, incubate and integrate this solution within their operations.